|



|
A Novel Fault Detection, Identification and Prediction Approach for Autonomous Vehicle Controllers Using SVM
Liam Biddle & Saber Fallah
|
|
|
Faults that develop in vehicle sensors have the potential to propagate unchecked throughout control systems if undetected. Automatic fault diagnosis and health monitoring algorithms will become necessary as automotive applications become more autonomous. The current fault diagnosis systems are not effective for complex systems such as autonomous cars where the case of simultaneous faults in different sensors is highly possible. Therefore, this paper proposes a novel fault detection, isolation and identification architecture for multi-fault in multi-sensor systems with an efficient computational burden for real-time implementation. Support Vector Machine techniques are used to detect and identify faults in sensors for autonomous vehicle control systems. In addition, to identify degrading performance in a sensor and predict the time at which a fault will occur, a novel predictive algorithm is proposed. The effectiveness and accuracy of the architecture in detecting and identifying multiple faults as well as the accuracy of the proposed predictive fault detection algorithm are verified through a MATLAB/IPG CarMaker co-simulation platform. The results present detection and identification accuracies of 94.94% and 97.01%, respectively, as well as a prediction accuracy of 75.35%.
|
|
Keywords: Fault detection · Fault identifcation · Fault prediction · Support Vector Machine
Biddle, L., Fallah, S. A Novel Fault Detection, Identification and Prediction Approach for Autonomous Vehicle Controllers Using SVM. Automot. Innov. 4, 301–314 (2021)
|
|
Full Paper Reading>>
|
|
|



|
End-to-End Autonomous Driving Through Dueling Double Deep Q-Network
Baiyu Peng, Qi Sun, Shengbo Eben Li, Dongsuk Kum, Yuming Yin, Junqing Wei & Tianyu Gu
|
|
|
Recent years have seen the rapid development of autonomous driving systems, which are typically designed in a hierarchical architecture or an end-to-end architecture. The hierarchical architecture is always complicated and hard to design, while the end-to-end architecture is more promising due to its simple structure. This paper puts forward an end-to-end autonomous driving method through a deep reinforcement learning algorithm Dueling Double Deep Q-Network, making it possible for the vehicle to learn end-to-end driving by itself. This paper firstly proposes an architecture for the end-to-end lane-keeping task. Unlike the traditional image-only state space, the presented state space is composed of both camera images and vehicle motion information. Then corresponding dueling neural network structure is introduced, which reduces the variance and improves sampling efficiency. Thirdly, the proposed method is applied to The Open Racing Car Simulator (TORCS) to demonstrate its great performance, where it surpasses human drivers. Finally, the saliency map of the neural network is visualized, which indicates the trained network drives by observing the lane lines. A video for the presented work is available online, https://youtu.be/76ciJmIHMD8 or https://v.youku.com/v_show/id_XNDM4ODc0MTM4NA==.html.
|
|
Keywords: End-to-end autonomous driving · Reinforcement learning · Deep Q-network · Neural network
Peng, B., Sun, Q., Li, S.E. et al. End-to-End Autonomous Driving Through Dueling Double Deep Q-Network. Automot. Innov. 4, 328–337 (2021)
|
|
Full Paper Reading>>
|
|
|



|
A Computer Graphics-Based Framework for 3D Pose Estimation of Pedestrians
Jisi Tang & Qing Zhou
|
|
|
In pedestrian-to-vehicle collision accidents, adapting safety measures ahead of time based on actual pose of pedestrians is one of the core objectives for integrated safety. It can significantly enhance the performance of passive safety system when active safety maneuvers fail to avoid accidents. This study proposes a deep learning model to estimate 3D pose of pedestrians from images. Since conventional pedestrian image datasets do not have available pose features to work with, a computer graphics-based (CG) framework is established to train the system with synthetic images. Biofidelic 3D meshes of standing males are first transformed into several walking poses, and then rendered as images from multiple view angles. Subsequently, a matrix of 50 anthropometries, 10 gaits and 12 views is built, in total of 6000 images. A two-branch convolutional neural network (CNN) was trained on the synthetic dataset. The model can simultaneously predict 16 joint landmarks and 14 joint angles of pedestrian for each image with high accuracy. Mean errors of the predictions are 0.54 pixels and − 0.06°, respectively. Any specific pose can then be completely reconstructed from the outputs. Overall, the current study has established a CG-based pipeline to generate photorealistic images with desired features for the training; it demonstrates the feasibility of leveraging CNN to estimate the pose of a walking pedestrian from synthesized images. The proposed framework provides a starting point for vehicles to infer pedestrian poses and then adapt protection measures accordingly for imminent impact to minimize pedestrian injuries.
|
|
Keywords: Pose estimation · Pedestrian safety · Computer graphics · Convolutional neural network
Tang, J., Zhou, Q. A Computer Graphics-Based Framework for 3D Pose Estimation of Pedestrians. Automot. Innov. 4, 338–349 (2021)
|
|
Full Paper Reading>>
|
|
|
|
|
|
|
Automotive Innovation 2020 Best Paper Award Announced
|
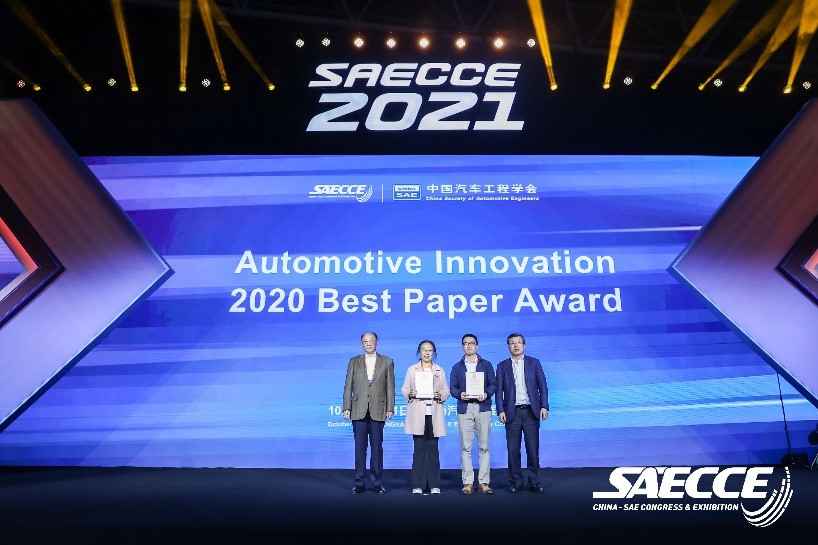
Automotive Innovation 2020 Best Paper Award was organized and the award ceremony was held in Shanghai during SAECCE 2021 on October 19, 2021. The objective of the annual AUIN Best Paper Award is to recognize outstanding contributions to the body of theoretical or practical knowledge in the automotive field. It is hoped that more engineers and researchers could publish their research in Automotive Innovation.
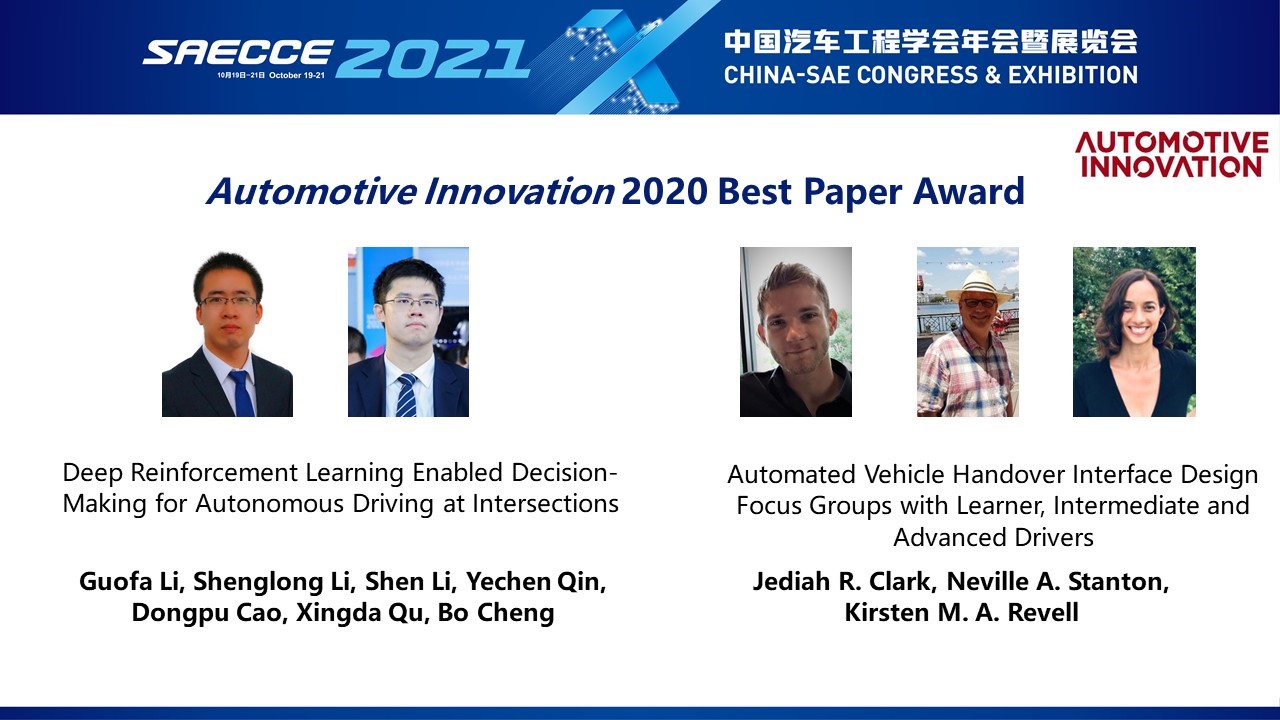
The first awarded paper:
Deep Reinforcement Learning Enabled Decision-Making for Autonomous Driving at Intersections
The second awarded paper:
Automated Vehicle Handover Interface Design Focus Groups with Learner, Intermediate and Advanced Drivers
For more information, please click here.
|
|
|
|
|
|
SAECCE 2021: Top 10 technology trends in China’s automotive industry for 2022 released
|
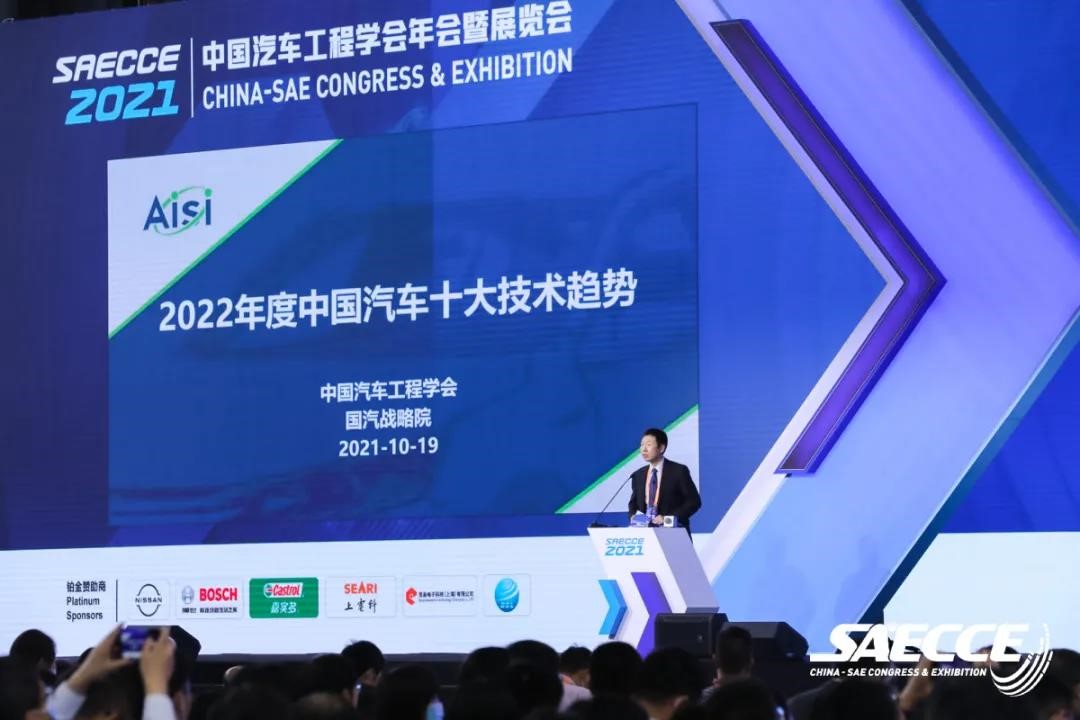
On October 19, China SAE released the Top 10 Technology Trends in China’s Automotive Industry for 2022 at the opening ceremony of SAECCE2021.
Top 10 Technology Trends in China’s Automotive Industry for 2022:
1. Mass production of computer chips with over 100TOPS will be achieved for automotive application.
2. Mass production of the third-generation semiconductor motor controller will be realized for OEMs.
3. Rising safety technology promotes the application of battery with high energy density of 300Wh/kg in vehicles.
4. Long-lifetime fuel cell system will be applied in commercial vehicles and under multiple working conditions.
5. Market share of pure electric passenger cars based on dedicated platform will exceed 65%.
6. High-performance pure electric passenger cars in China will adopt more 800V high-voltage platforms.
7. Intelligent thermal management technology will significantly improve the low-temperature adaptability of new energy vehicles.
8. Domain controller will realize the breakthrough in transition technology from single-domain control to cross-domain fusion control.
9. Automotive cybersecurity technology will realize great development from border defense to active deep defense.
10. Installation of DHT system on vehicles will be multiplied.
Please click here to find out more.
|
|
|
|
|
|
FISITA World Mobility Summit to be held on Oct.20 & Nov. 11
|
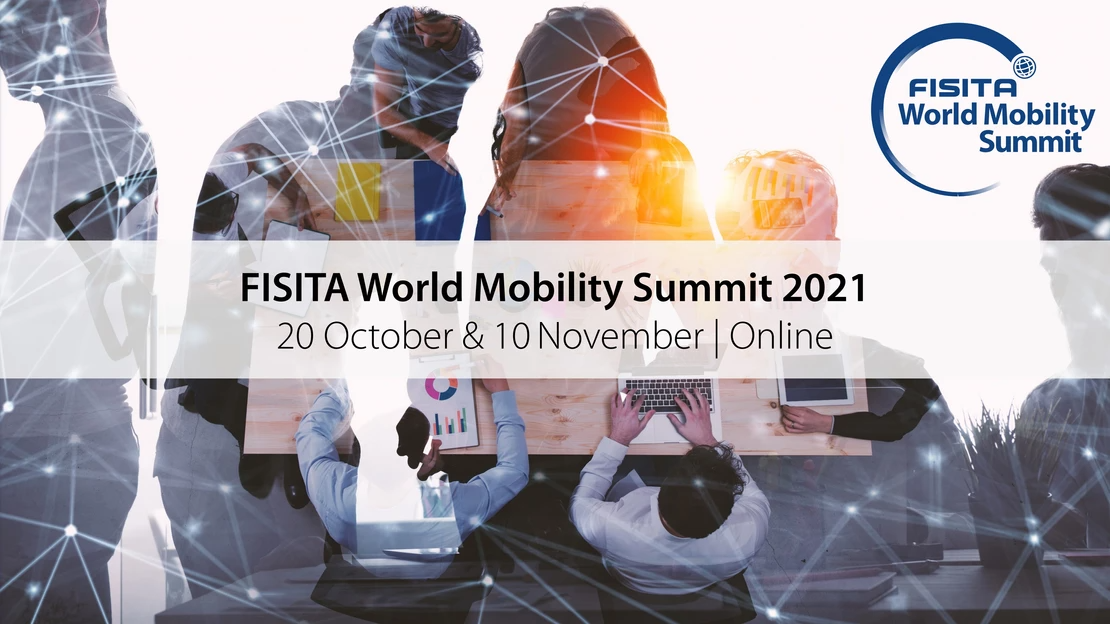
The FISITA World Mobility Summit is a unique and exclusive annual opportunity which brings together the technical leaders of the FISITA Corporate Membership and invited guests. The Summit will commence with the ‘World Café' discussion sessions to be held on October 20th, enabling registered guests to come together virtually and share their initial thoughts on the challenges and opportunities of ‘Workplace Evolution’, with outcomes being created and delivered as part of the Summit agenda on 10 November.
Throughout the Summit we will explore how the business community is and will evolve, what are the challenges and opportunities within the workplace, how will our people be motivated and what do we anticipate will be the most significant impacts on the long-term change in human behaviour.
Further details about the Summit can be found here.
|
|
|
Automotive Innovation
Sponsored by China SAE and published globally via Springer Nature, Automotive Innovation aims to be a world-class journal that provides abundant sources of innovative findings for automotive engineers and scientists. The journal is published quarterly, ensuring high-quality papers satisfying international standards. With the editorial board consisting of world-renowned experts, it has attracted readers from 72 countries and regions. The highest download of a single article wins more than 18,000.
The journal provides a forum for the research of principles, methodologies, designs, theoretical background, and cutting-edge technologies in connection with the development of vehicle and mobility. The main topics cover: energy-saving, electrification, intelligent and connected, safety, and emerging vehicle technologies.
Editors-in-Chief
Jun Li, Academician of CAE, President of China SAE, Professor of Tsinghua University
Frank Zhao, President (2018-2020) of FISITA, President of Tsinghua Automotive Strategy Research Institute
Executive Editor-in-Chief
Prof. Mike Ma,Executive Chief Editor of Automotive Innovation, Professor of Jilin University, VP Technical FISITA
|
|
Paper submission and browse
www.ChinaSAEJournal.com.cn
www.springer.com/42154
Contacts:
Ms. Lily Lu
Tel: +86-10-50950101
Email: jai@sae-china.org
|
|
Sponsored by
|
Published by
|
|