If you can't browse the mail properly, please
click here
|
中文版, 请
点击这里
|
|
Free Submission & Reading>>
|
 |
|
The journal Automotive Innovation is sponsored by China SAE,
published through Springer Nature, distributed around the
world, it reflects the top-level research and technological
advance of automotive engineering.
Automotive Innovation newsletter in October includes the
following contents:
1. Article Recommendation——Three papers on Autonomous
Vehicles
2. China SAE News:
- Automotive Innovation 2022 Best Paper Award Announced
- SMC 2023 to be held on Nov. 15-16 in Shanghai
-
2023 Formula Student Combustion China successfully held in
Xiangyang, China
|
|
|
 |
|



|
A Bayesian Approach with Prior Mixed Strategy Nash
Equilibrium for Vehicle Intention Prediction
Giovanni Lucente, Reza Dariani, Julian Schindler, Michael
Ortgiese
|
|
|
The state-of-the-art technology in the field of vehicle
automation will lead to a mixed traffic environment in the
coming years, where connected and automated vehicles have to
interact with human-driven vehicles. In this context, it is
necessary to have intention prediction models with the
capability of forecasting how the traffic scenario is going to
evolve with respect to the physical state of vehicles, the
possible maneuvers and the interactions between traffic
participants within the seconds to come. This article presents a
Bayesian approach for vehicle intention forecasting, utilizing a
game-theoretic framework in the form of a Mixed Strategy Nash
Equilibrium (MSNE) as a prior estimate to model the reciprocal
influence between traffic participants. The likelihood is then
computed based on the Kullback-Leibler divergence. The game is
modeled as a static nonzero-sum polymatrix game with individual
preferences, a well known strategic game. Finding the MSNE for
these games is in the PPAD \cap PLS complexity class, with
polynomial-time tractability. The approach shows good results in
simulations in the long term horizon (10s), with its
computational complexity allowing for online applications.
|
|
Keywords: Vehicle intention prediction, Trajectory prediction,
Bayesian approach, Mixed strategy, Nash equilibrium
Lucente, G., Dariani, R., Schindler, J. et al.: A Bayesian
Approach with Prior Mixed Strategy Nash Equilibrium for
Vehicle Intention Prediction. Automot. Innov. 6, 425–437
(2023)
|
|
Full Paper Reading>>
|
|
|



|
Deep Reinforcement Learning Based Decision-Making Strategy of
Autonomous Vehicle in Highway Uncertain Driving Environments
Huifan Deng, Youqun Zhao, Qiuwei Wang, Anh-Tu Nguyen
|
|
|
Uncertain environment on multi-lane highway, e.g., the
stochastic lane-change maneuver of surrounding vehicles, is a
big challenge for achieving safe automated highway driving. To
improve the driving safety, a heuristic reinforcement learning
decision-making framework with integrated risk assessment is
proposed. First, the framework includes a long short-term memory
model to predict the trajectory of surrounding vehicles and a
future integrated risk assessment model to estimate the possible
driving risk. Second, a heuristic decaying state entropy deep
reinforcement learning algorithm is introduced to address the
exploration and exploitation dilemma of reinforcement learning.
Finally, the framework also includes a rule-based vehicle
decision model for interaction decision problems with
surrounding vehicles. The proposed framework is validated in
both low-density and high-density traffic scenarios. The results
show that the traffic efficiency and vehicle safety are both
improved compared to the common dueling double deep Q-Network
method and rule-based method.
|
|
Keywords: Automated driving, Decision making, Uncertain driving
environments, Reinforcement learning, Multi-lane traffic,
Integrated risk assessment
Deng, H., Zhao, Y., Wang, Q. et al.: Deep Reinforcement
Learning Based Decision-Making Strategy of Autonomous Vehicle
in Highway Uncertain Driving Environments. Automot. Innov. 6,
438–452 (2023)
|
|
Full Paper Reading>>
|
|
|



|
On-ramp Merging for Highway Autonomous Driving based on Deep
Reinforcement Learning
Guofa Li, Weiyan Zhou, Shen Li, Xingda Qu
|
|
|
This paper proposes an improved decision-making method based on
deep reinforcement learning to address on-ramp merging
challenges in highway autonomous driving. A novel safety
indicator, time difference to merging (TDTM), is introduced,
which is used in conjunction with the classic time to collision
(TTC) indicator to evaluate driving safety and assist the
merging vehicle in finding a suitable gap in traffic, thereby
enhancing driving safety. The training of an autonomous driving
agent is performed using the Deep Deterministic Policy Gradient
(DDPG) algorithm. An action-masking mechanism is deployed to
prevent unsafe actions during the policy exploration phase. The
proposed DDPG + TDTM + TTC solution is tested in on-ramp merging
scenarios with different driving speeds in SUMO and achieves a
success rate of 99.96% without significantly impacting traffic
efficiency on the main road. The results demonstrate that
DDPG + TDTM + TTC achieved a higher on-ramp merging success rate
of 99.96% compared to DDPG + TTC and DDPG.
|
|
Keywords: Autonomous driving, On-ramp merging, Deep
reinforcement learning, Action-masking mechanism, Deep
Deterministic Policy Gradient (DDPG)
Li, G., Zhou, W., Lin, S. et al. On-Ramp Merging for Highway
Autonomous Driving: An Application of a New Safety Indicator
in Deep Reinforcement Learning. Automot. Innov. 6, 453–465
(2023)
|
|
Full Paper Reading>>
|
|
|
 |
|
|
|
|
Automotive Innovation 2022 Best Paper Award Announced
|
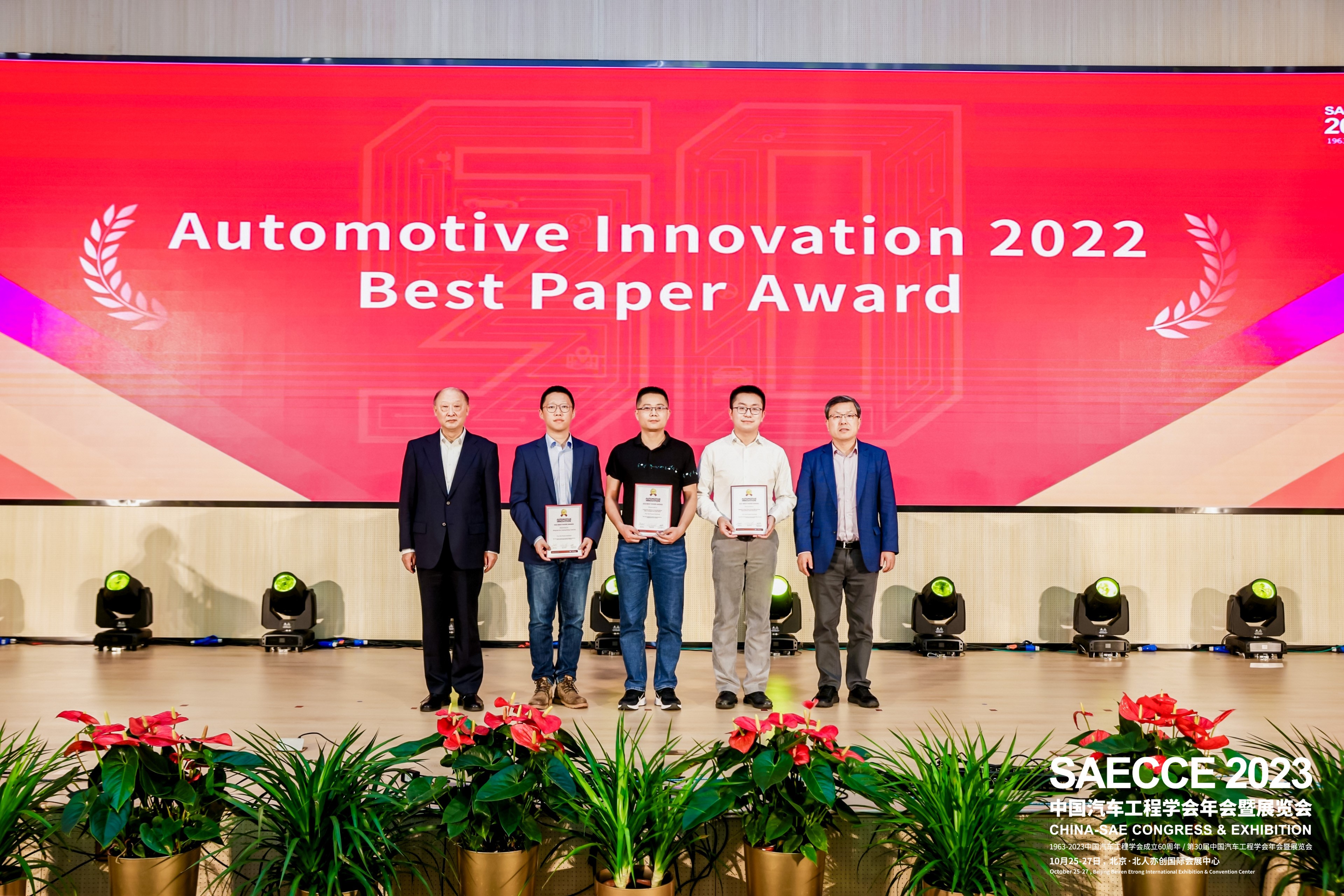
Automotive Innovation 2022 Best Paper Award was organized. The
objective of the annual AUIN Best Paper Award is to recognize
outstanding contributions to the body of theoretical or
practical knowledge in the automotive field.
The Award was selected by the Award Committee based on general
quality of paper, subject matter, originality, scientific,
technological and innovative excellence of the paper, as well
as the download and citation. 3 best papers were selected from
34 articles published in Automotive Innovation in 2022:
-
Real-Time Predictive Control of Path Following to
Stabilize Autonomous Electric Vehicles Under Extreme Drive
Conditions(Authors: Ningyuan Guo, Xudong Zhang, Yuan
Zou)
-
Cyber Hierarchy Multiscale Integrated Energy Management
of Intelligent Hybrid Electric Vehicles(Authors: Yanfei
Gao, Shichun Yang, Xibo Wang, Wei Li, Qinggao Hou, Qin
Cheng)
-
Internal Short Circuit Detection for Parallel-Connected
Battery Cells Using Convolutional Neural Network(Authors:
Niankai Yang, Ziyou Song, Mohammad Reza Amini, Heath
Hofmann)
|
|
|
|
|
|
SMC 2023 to be held on Nov. 15-16 in Shanghai
|
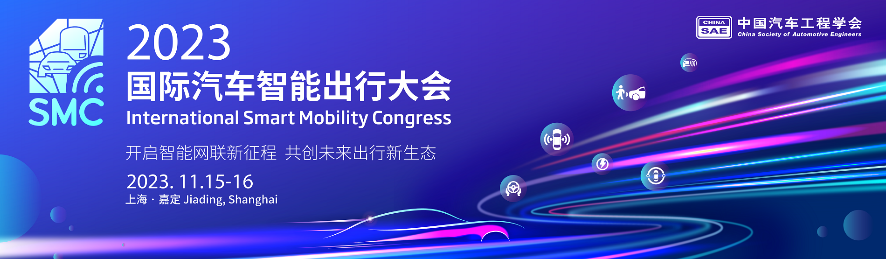
China SAE will host the 4th International Smart Mobility
Congress on November 15-16, 2023 in Shanghai, to jointly promote
the integration and international cooperation of the intelligent
and connected vehicle industry. The congress contains 1 main
forum, 5 technical sessions, covering over 100 speakers and over
300 companies.
For more detailed information about the conference and
exhibition, click
here
.
|
|
|
|
|
|
2023 Formula Student Combustion China successfully held in
Xiangyang, China
|
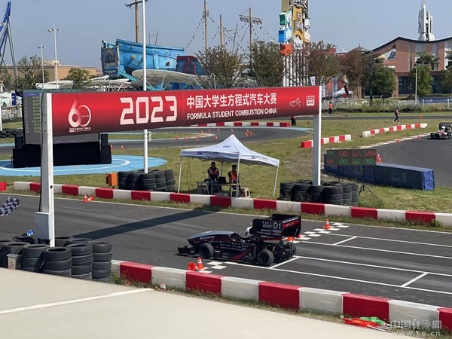
On October 14, the 2023 Formula Student Combustion China came to
a successful close. This year's competition featured three
static events, including design defense, marketing defense, and
cost defense; and five dynamic events, including straight-line
acceleration, figure-of-eight loop, high-speed obstacle
avoidance, endurance race, and efficiency test. A total of 56
teams registered for this year's competition, and there were
over 2,000 participants. After four days of intense competition,
Jilin University emerged as the champion in this year's Formula
Student Combustion China, securing a total score of 900.86. The
second place was claimed by Xiamen Institute of Technology with
a score of 870.51, while Liuzhou Institute of Technology secured
the third place with a score of 853.06.
For more information, please click
here
.
|
|
|
 |
|
Automotive Innovation
Sponsored by China SAE and published globally via Springer
Nature, Automotive Innovation aims to be a world-class journal
that provides abundant sources of innovative findings for
automotive engineers and scientists. The journal is published
quarterly, ensuring high-quality papers satisfying international
standards. With the editorial board consisting of world-renowned
experts, it has attracted readers from 72 countries and regions.
The highest download of a single article wins more than 32,000.
The journal is indexed in Ei Compendex, ESCI, and Scopus
(IF2022=6.1).
The journal provides a forum for the research of principles,
methodologies, designs, theoretical background, and cutting-edge
technologies in connection with the development of vehicle and
mobility. The main topics cover: energy-saving, electrification,
intelligent and connected, safety, and emerging vehicle
technologies.
Editors-in-Chief
Jun Li, Academician of CAE, President of China SAE, Professor of
Tsinghua University
Frank Zhao, Honorary Lifetime President of FISITA, Director of
Tsinghua Automotive Strategy Research Institute, Professor of
Tsinghua University
Executive Associate Editor-in-Chief
Prof. Xinjie Zhang, Professor of Jilin University
|
|
Paper submission and browse
www.ChinaSAEJournal.com.cn
www.springer.com/42154
Contacts:
Ms. Lily Lu
Tel: +86-10-50950101
Email:
jai@sae-china.org
|
|
Sponsored by
|
Published by
|
|